Demystifying the business of AI, machine learning, big data…
Andrew Wu discusses more emerging technologies that make the investment process more efficient and more accessible. Wu talks about misconceptions surrounding AI, big data and machine learning within investment technology.
Excerpt From
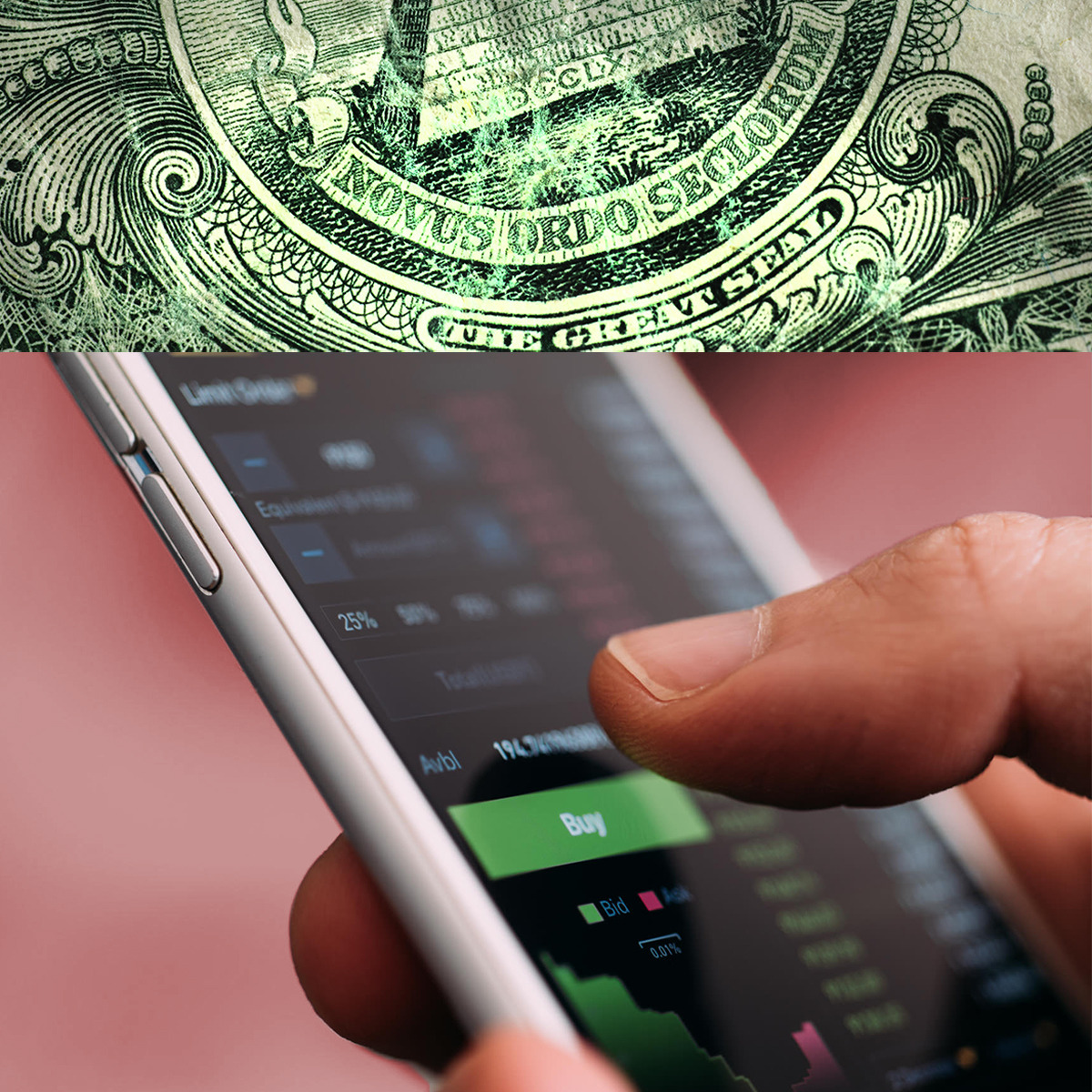
Transcript
The tech in the investec sector
really comes in two flavors. There are a lot of new technologies that
make the investment process more efficient and more accessible. We've seen some of that in
the first module of this course. Automation in asset allocation, financial
planning and security settlement have really started to drive down cost
across many investment sectors and enable new developments like Robo
advisers, automated financial planners and smart index funds to make the average
investors portfolios more customized and more diversified and
at the same time less costly. The other category are the technologies
that make the investment process more precise and these are broadly
labeled sometimes incorrectly as AI, big data and
machine learning technologies, which are simply data analytics tools
that enhance our ability to interpret the historical financial data and make
more accurate predictions for the future. We've talked about some of
these such as neural networks primarily from a technical
point of view in this. Module will take a broader business focus
point of view on the application of AI and machine learning techniques
in the investment industry. Will take a primarily non-technical
approach in this module with the goal of providing an industry overview did
mystifying the key buzzwords and clarifying some common misconceptions
providing some practical advice and directing you to the more
in-depth research and analysis in the relevant subject
fields such as computer science. In statistics, should you be
interested in pursuing further? First let's take a look at what Ai and or machine learning really
are as these concepts are probably on par with crypto as some
of the most over hyped terms in finance. First of all, there are two types of
AI systems one is called narrow AI which is defined by divorce key is
a computer system that matches or exceeds human intelligence, but
in a narrowly defined area under this definition most computer programs can
be called narrow AI to some degree as most of them would exceed human's
capabilities in some area next. The general AI is probably the one
that you're more familiar with and that's essentially
The Skynet is a software. But without the pre-program rules
an artificial neural network that is truly able to code itself and
rewire self with new data patterns. Essentially the general AI is a true
imitation of the human brain so far, the current computing
capabilities of our hardware is far from being able to even remotely
construct a general AI network. So most of the research in this
type has been theoretical therefore the current application of all of
the so-called AI systems in finance has been at best narrow AI, a more
appropriate label for AI in finance though is probably machine learning you see
the financial industry particularly. The investment sector has been
all about processing data and information extracting new insights and
signals from current and historical data. In these settings the use of AI has
essentially been the use of more and more advanced models of machine learning. Which is self is a fancy buzzword for
statistics essentially using machine learning or quote unquote AI investments
is using advanced statistical models on the same financial data to
better interpret patterns in the data conduct better statistical inferences or
make better predictions. Now, let's put the tools and
the data together. To have a more complete picture of
the type of the data out there in the investment landscape and the type of
tools that you can use on them first. There are all kinds of data that you can
use to guide your investment decisions out there numbers, reports,
text, news, you name it. Now, these data will belong
to one of two types. Let's call the first type
quote-unquote small data. These are the data small
enough in size like files and excel spreadsheets that you can store. Or on your computer and
search on your computer and the counterpart to that is big data. And what's Big Data? Well data set that's large enough that you
can't store it on your own computer, but instead have to put it in
a dedicated storage server and have to use some specialized
database tools like Hadoop and Mapreduce to efficiently process and
manage the data. That's it. That's literally the bus free meaning of
big data now within both the small data. And dictate the categories data can
further be classified into two types based on their structure. The first type is called structured data. These data are essentially numbers,
numbers that you can organize neatly in rows and columns like in
an excel spreadsheet now surprisingly. It's a little easier to perform
analysis on structured data because there are already quite organized
in contrast to that are unstructured data. These are the natural languages,
text, images, videos, etc. Because these data are not neatly
stored as numbers in rows and columns that will take a little
bit more effort to analyze first. We'll have to use some statistical
technique like natural language processing or NLP for text data and
signal analysis for audio and video data to convert these data into
numbers before we can analyze them but the important thing to note here, is that
contrary to what you hear in the hype. There's no mutual exclusivity
between the data types. For example, not all unstructured
data are big data things like text or image files could be very small in size. So you wouldn't need mapreduce
to work with them, but they still require the extra processing
step to be converted into numbers. There could be small unstructured data and there could be big structured data,
now with all that data available. Here are all the tools that you can use
to mine these data for insights and signals starting with the simplest. We have what I call the simple analytics
like basic summary statistics, for example, averages standard
deviations correlations etc. Now basically everything else can
be labeled as machine learning which again is just a fancy buzzword for
the more advanced statistical models beyond simple statistics, like averages
that you can use on the data to analyze patterns conduct inferences or
make predictions next. There are two types of machine
learning tools the easier type. Let's call that quote unquote shallow
learning a classic example of shallow machine learning is a linear regression,
shallow learning is essentially a statistical model where we the user have
to specify most of the model parameters. For example,
when you're estimating a regression, you have to choose what
are the independent variables the regressors that you want
to include in that regression. The counterpart to that
is deep learning and the bus free meaning of deep learning is
essentially a statistical model with more parameters that can be directly determined
by the data an example of deep learning is regularized linear regressions,
like the lasso or the elastic net. These are the regression
models where instead of you choosing the independent variables you low
the entire variable list to the model and based on some basic criteria. The model will choose the most quoted. Quote important regressors to include for
you that's essentially deep learning, letting the data determine the model
parameters from the last module. We already saw that neural networks or
another classic example of deep learning. And this class of models is the closest to
quote unquote and AI that we can use for financial data analytics and
further within each machine learning type. We also have two sub categories. The first category is called
supervised learning and as its name suggests supervised learning
models have to be trained with data before we can use them for
tasks like predictions again, a linear regression is a classic
example of supervised machine learning. Or lending regression examples
from the credit tech class any regression model there has
to be fitted with data first. We need to get the parameter estimates for
these alphas and betas and only then can we plug in new data and get the predicted values like the default
rates by this token, you can view other much more advanced supervised learning
models as super souped-up regressions. The other class of machine
learning models are unsupervised. This means that we don't need to use
existing data to estimate the model before we can use it. We can just directly use it a classic
example of unsupervised learning models is clustering given
a bunch of data points. You can directly take them to
a clustering model and the model will cluster them into groups where
points within a group are close enough. We've seen a model like that in
action in the platform landing module of the credit tech course, so
basically both shallow learning and deep learning can either be supervised or unsupervised finally to put the data and
the analytics together. Well, you have complete freedom to
choose whatever tool you see fit to analyze the type of data at hand. You can use a supervised shallow
learning tool like a regression to analyze structured big data and there
are unsupervised deep learning tools for unstructured small data when it comes
to using AI or machine learning for investments. You're really limited by number one. Your computing power and number two
choosing the most appropriate model that works best for
your data type and your questions. Let's now take a deeper look at the usage
of unstructured data in investments because with the competition for
Alpha is getting more and more intense. Investors are increasingly turning to the
analysis of unstructured financial data under using ever more
sophisticated models to mine them, if you think about it in finance the bulk
of the growth in data that we have available has been an unstructured data. This is from Apple's SEC 10K
filing is annual report for 2019. And this income statement here is
an example of structured data with numbers neatly stored in columns for
tabulation and analysis. And when released these data are obviously
the first focus of analysts and traders alike. However, think about how long
this annual statement is the tables are only
a few pages at best and the rest of the statement that hundreds of
pages of text is all unstructured data. Much fewer people pay attention to
these not to mention finishing them as they're not very exciting reading but these languages might
contain a lot of information. What worse and what sentences are used and how they're put together can tell
you a lot about what the management might be thinking beyond just the income
numbers, same for the social media data. This is a random Twitter page with posts
about Apple stocks at the end of 2019. As you can see these social
media languages how positive or uncertain they are for example can also
tell us a lot about investor sentiment and psychology around the stock. Of course, there's way too much
of these unstructured data text images that are relevant to the stock
out there for us to read them all and that's precisely where computers can help. For example,
we can use natural processing tools to first convert the text
into some form of numbers that we can fit our existing machine
learning models on at the simplest level. For example, the whole 10 K filing could
be turned into a word vector with entries denoting to the frequencies of each
English word that's used in the document. We can then stack different document
vectors together into a matrix and run all sorts of shallow and deep machine learning
algorithms on it to get an estimate for both content and on text like how
positive the languages are how complex the sentence structure is how many topics
are talked about among many other things. So I encourage you to explore a bit more
about natural language processing and other methods for
unstructured data analysis, because when used appropriately they can
really extract information and signals from these data that are truly incremental
to what you get from purely the numbers.