Producing More, Understanding Less
In this video, Professor Patrick Barry explores the role of AI in education and leadership, examining its potential to enhance creativity, personalize learning, and support skill development. Throughout the video, you will engage with diverse perspectives on AI's benefits and challenges, including concerns about intellectual homogenization and the importance of using AI responsibly to foster innovation and adaptability.
Excerpt From
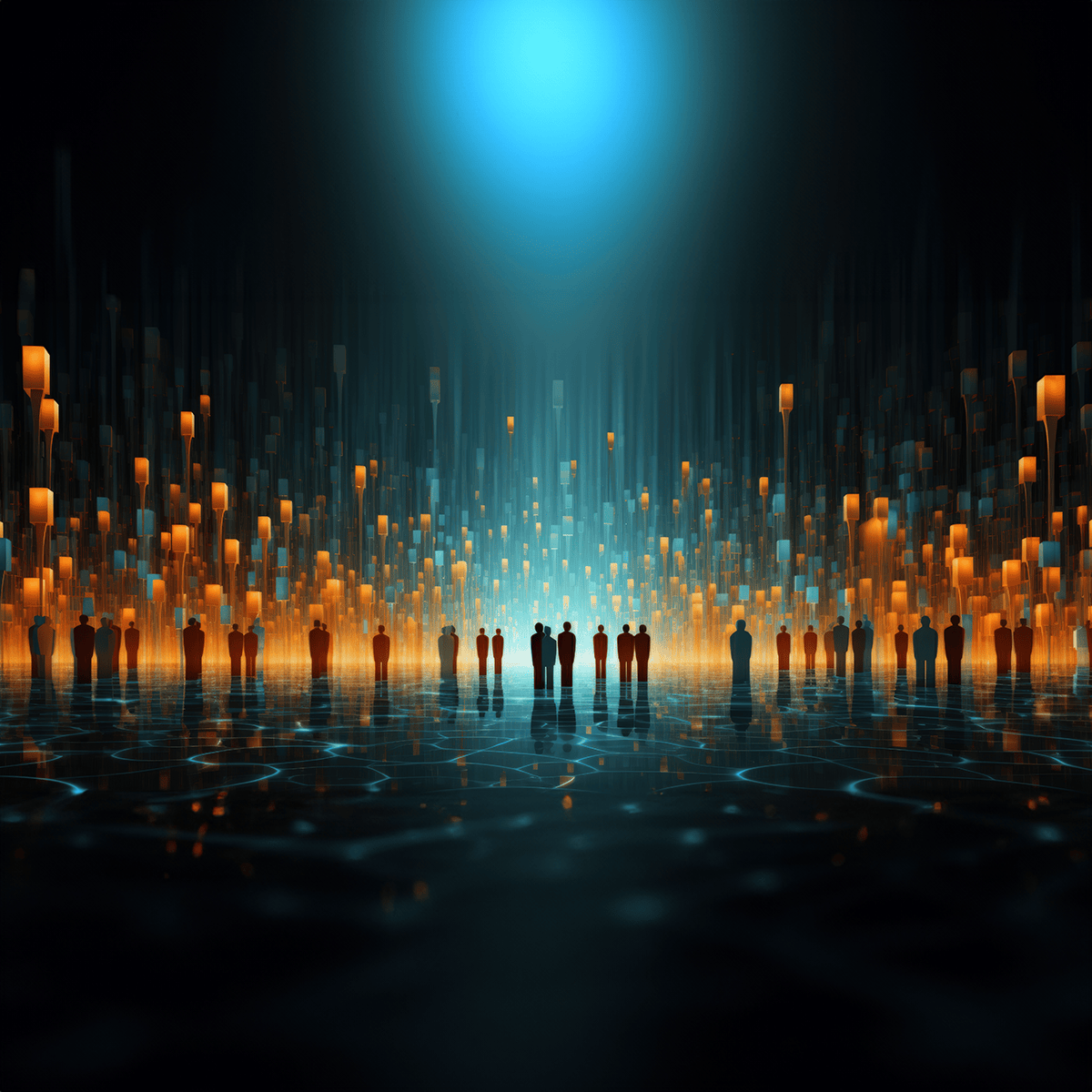
Transcript
We ended the previous course
in our AI for lawyers and other advocate series
by grappling with the problem of
delegation remorse. That feeling of
regret that comes when you realize the
task, assignment, or activity you delegated away is actually something you
wish you could keep doing. The initial example we used came from Economist
Steve Levitt, the co-author of Freakonomics, and a past recipient of the
Prestigious Bates Medal, which is given to the
top American economist under the age of 40. Here's a reminder of
how Levitt articulated the delegation remorse
he experienced after. In an effort to become more
efficient as a scholar, he began to delegate to
his research assistants, the extremely time-consuming, but also to Levitt, very joy-producing
task of data analysis. I ended up delegating
away what I loved in pursuit of
higher productivity. In the end, I was
productive, but honestly, all the fun went out of it, and I soured on
academic research. A related concern comes in the paper by
the anthropologist Lisa Messeri and
the neuroscientist Michelle Crockett, we
mentioned in course two. They're the ones who worry
that an overdependence on AI, particularly among scientists
might lead us to a world in which we produce more
but understand less. Messeri and Crockett
are not AI Domers, early on in the paper, which was published in
the journal Nature, under the title Artificial
Intelligence and Illusions of Understanding
and Scientific Research they make clear that we do
not take the position that AI should never be used
in scientific research. Soon after the paper was
published, Crockett, who specializes in
machine learning and natural language processing, told the reporter at the Science Magazine
ARS Technica that I have used AI in my work, and I'm really excited
about its potential. Yet both Crockett and Messeri, nevertheless worry about
what may happen should AI-assisted research come to dominate the production
of scientific knowledge. One of their particular fears is a situation where because of the seductive convenience
and efficiency of AI tools, more and more people rely on these tools for more and more
of the research process. Reading, summarizing, and analyzing existing
data and information, generating new ideas
and hypotheses, describing, editing, and
disseminating findings. This intellectual
homogenization might result in a scientific
monoculture that lacks crucial elements
of creativity and diversity and is
vulnerable to error, bias and missed opportunities
for innovation. In other words,
if everybody uses the same co-author
and co-brainstormer, and co-editor, we could find ourselves with a
dangerously narrow, stagnant, and incomplete
understanding of the world. Messeri and Crockett's
misgivings may remind you of a few of the red flags raised by somebody else we talked
about in course 2, Ethan Mollick, a business
professor at Wharton, and the author of
Co-Intelligence, living and working with AI. Here, as a review, is how he explained
the risk that AI's alluring ease might have a stifling effect on invention
and original thinking. The implication of having
AI write our first drafts. Even if we do the
work ourselves, which is not a given are huge. One consequence is
that we could lose our creativity and originality. When we use AI to generate
our first drafts, we tend to anchor on the first idea that
the machine produces, which influences
our future work. Even if we rewrite the
drafts completely, they will still be tainted
by the AI's influence. We describe this process
as a path dependence. We're starting out in
a certain direction constrains the next moves
you might decide to take. If we rely on AI to set our
initial options for us, we miss out on the
chance to explore different perspectives and
alternatives, Mollick writes. Some of which may lead to much better solutions
and insights. We'll want to keep
these considerations in mind throughout this course, as we turn our attention
to the role AI may have and already has had an
education and leadership. How should teachers approach AI? How should students approach AI? How about the leaders
of law firms, government agencies, and
nonprofit organizations, all of whom know that a key driver of
success is making sure their employees have the skills necessary to thrive in
a fast-changing world? Many like Sal Khan, the founder of the Online Education Powerhouse
Khan Academy are incredibly
optimistic about AI. In brave new words, how AI will revolutionize education and why that's
a good thing he details how Khan Academy has used AI to create a
platform that offers every person an
opportunity to engage deeply in the education
process in entirely new ways. Among other things, it provides a personalized and patient
tutor that focuses on the learners' interests
or struggles and empowers educators to better understand how they can fully
support their students. There's also the
book Teaching with AI by Jose Antonio Bowen, a former president
of Goucher College, and C Edward Watson, the current Vice President
for Digital Innovation at the Association of American
Colleges and Universities. While acknowledging that, AI is already increasing inequity, both in education and beyond. They also make a case for its potential as a
powerfully beneficial tool. It can increase
human creativity and customize materials for groups
or individual students. Others like Dan Meyer, who earned his doctor in math education from
Stanford and later became the chief
academic officer at the Online Learning
Company Desmos, are more apprehensive, particularly when it comes
to chatbot pedagogy. Generative AI is best he has written at what something
teachers need least, Namely personalizing the text of a word problem to fit
a student's interest. He then cites multiple
studies that suggest that this kind of superficial intervention, where,
for instance, the math questions given to a student who likes basketball, will include examples
about basketball, and the math questions given to a student who likes puppies, will include examples about puppies don't really
work that well. You'll get to engage with these and other perspectives throughout the rest
of the course, beginning with one
I try to convey, whenever I talk about artificial intelligence,
with students, lawyers, teachers, administrators,
government officials, and pretty much anyone else. AI, when used well, can be an incredible
feedback machine.