Day in the Office: Before & After Generative AI
Generative AI has the possibility to make workflow processes more efficient and personalized. In this video, Professor Andrew Wu explores how generative AI is transforming business operations by streamlining tasks like email management, data analysis, marketing strategy, and customer service.
Excerpt From
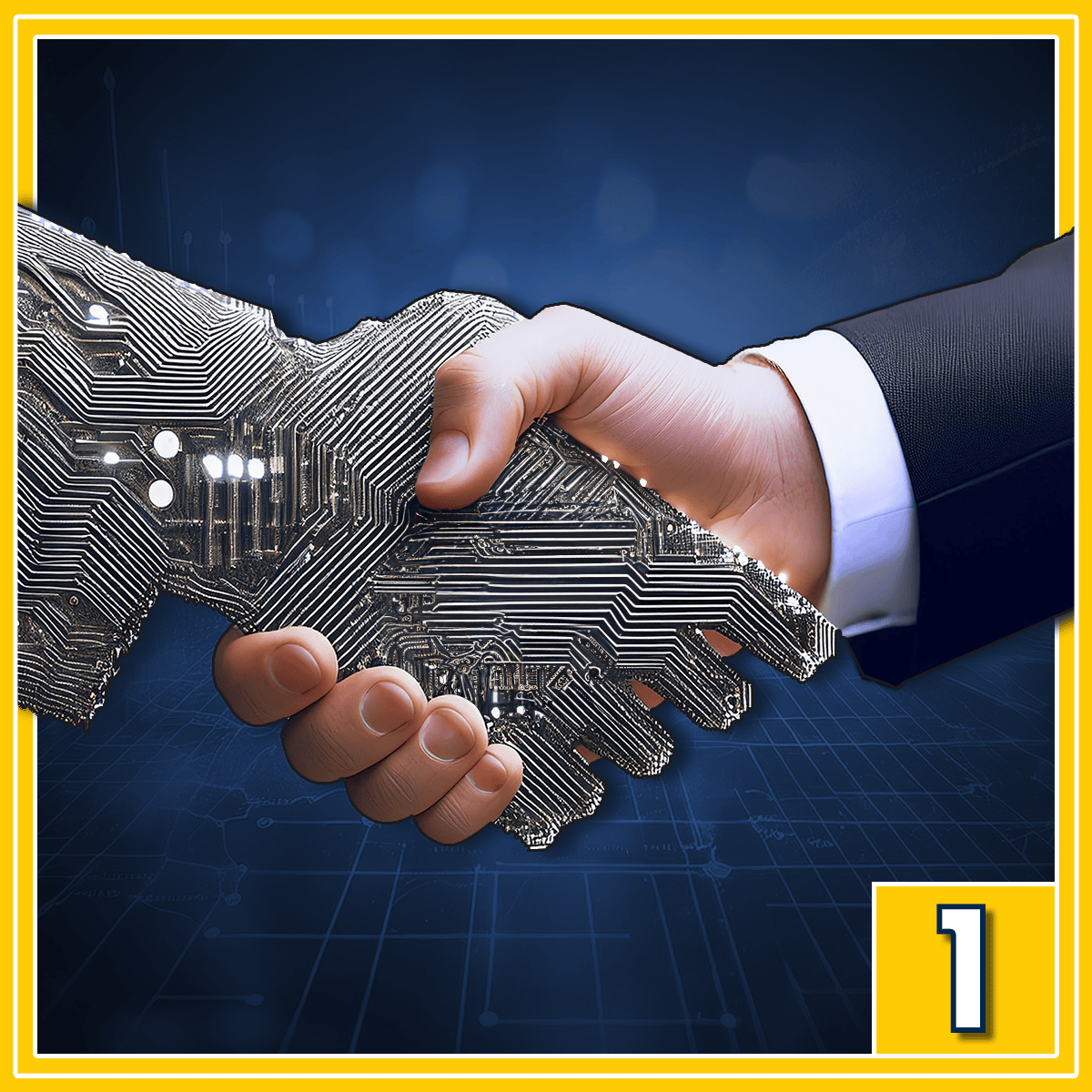
Transcript
Well, for many of us, our morning routine
in the office begins with the tried and true tradition
of checking our emails. Nothing remarkable about that. But as some of you might
have seen already, this practice is changing, for the better,
with generative AI. With AI copilots directly integrated into your
favorite email platform, our one hour routine of sorting
our inbox, prioritizing, and crafting responses could
be done in a matter of minutes with the help of
these copilot assistants. These tools are based on
large language models that absorb the emails that
you've written in the past to learn
how you communicate. After learning from these data, the AI copilot can
sort your emails, create drafts for
your responses, and even suggest the right
tone and style according to the audience all based on
your own writing style. All you need to do is
to provide it with a simple description of
what you want. Pretty cool. After your morning meeting, maybe it's time to do
some data analytics for the project that
you'll be working on. While you can have the intern in your data science team to do all the number
crunching for you, generative AI is rapidly
entering the workflow as well. For example, even tools like ChatGPT now possess sophisticated data
analytics capabilities. Instead of you having to code
in a programming language, you can now simply upload
your data into the AI model, tell the tool what you want to do with the data
in plain language, and you'll have the results. Just like in a
programming language, you can generate reports, create compelling data
visualizations and trend analysis, and even invoke very
sophisticated statistical models, all without needing a
technical background. The AI can convert your command into code and run it
for you directly. In other words, right now, gen AI is rapidly democratizing access to sophisticated
analytics. Therefore, allowing
more people to make data-driven informed
decisions faster, with greater confidence,
and potentially with no need for any
sophisticated programming. This could essentially
make any business professional a data
scientist at the same time. As the day progresses, let's say your analysis revealed a new consumer segment that
your company should target. Let's get that focus group
ready and start designing a new marketing
campaign. Wait a minute. Why not first simulating these campaigns using
generative AI so that you can try out your
marketing strategies before incurring any cost in
recruiting human subjects? This is going to be
a reality very soon. Say your company has done
marketing campaigns, focus groups,
surveys in the past. Then you can let a generative
AI model learn from these data and simulate the likely responses from
your target customers. With that, you can create a whole bunch of marketing
contents in one go, test them on your
simulated customers, predict their responses, segment them into priority list, and even design keywords for
search engine optimization. This could help you
target and retain specific audiences
more effectively with much lower cost, thereby making your
marketing campaigns and strategies much
more efficient. Of course, when it's time to actually deal with
these customers, generative AI can be a very effective companion to your traditional team
of human agents. Did you save all
these chat logs from your customer
service center? Now these conversation
histories can be put to use. Generative AI-based
customer service assistant tools can absorb these data and learn from them the best practices your team has built in dealing
with your customers. Armed with these insights
learned from your own data, these assistant
agents can help with your human agents process a larger volume of
customer inquiries. For example, before
your human agent even sees a chat
from a customer, the AI would have already prepared all the information
about their orders, preferences, and
past interaction histories for your agent. Then you can predict
their needs and provide personalized responses
for your human agent to use or edit. By taking over the
lower level processing, this frees up more time for your human agents to deal
with the more complex issues, ultimately enhancing
customer satisfaction. Finally, you can even leverage
generative AI to help you and your workforce with high level, even
strategic tasks. Everything that we've
talked about so far leaves a data trail, so don't let that go to waste. Generative AI can learn from these data and synthesize them into comprehensive reports, highlighting key insights and trends that might have
been missed otherwise. Many of the new tools can now search the Internet
directly as well to actively seek new
information and then package them into new insights on everything from
weather patterns, market conditions,
the macroeconomy, to supply chain risks. The end result is
that all of a sudden, your team now have a much
bigger repository of on-demand insights that you
can use to further make your business more
efficient and resilient. Hopefully, you saw that
generative AI is not just a mere chatbot that you use to tell stories
and search recipes. It can be a transformative force that touches every
part of a business. From strategy and operations to marketing, finance,
compliance, R&D, and customer service, generative AI can be used to
further drive efficiency, personalization, and innovation
on all of these fronts. It's even used to create
this presentation. However, to actually get
here is not a easy task. There's a very big wedge
between ChatGPT the chatbot, to a powerful business
asset that you can trust to be a productive
part of your organization. So, how do we get from here to here? Pause and think about
that for a moment. What is the common ingredient underneath all these new possibilities that
we talked about? What are the
additional ingredients that we need to inject into the chatbot to give it all these awesome
additional capabilities? Keep that in mind
and stay tuned.