Data Acquisition and GenAI
Ever wonder how to go from a big question to meaningful data? Professor Tina Lasisi breaks down how data is recorded and collected, reminding us to think carefully about its origins and accuracy. Using generative AI to brainstorm prompts and identify data sources, you can improve the way you gather and analyze information to answer real-world questions effectively.
Excerpt From
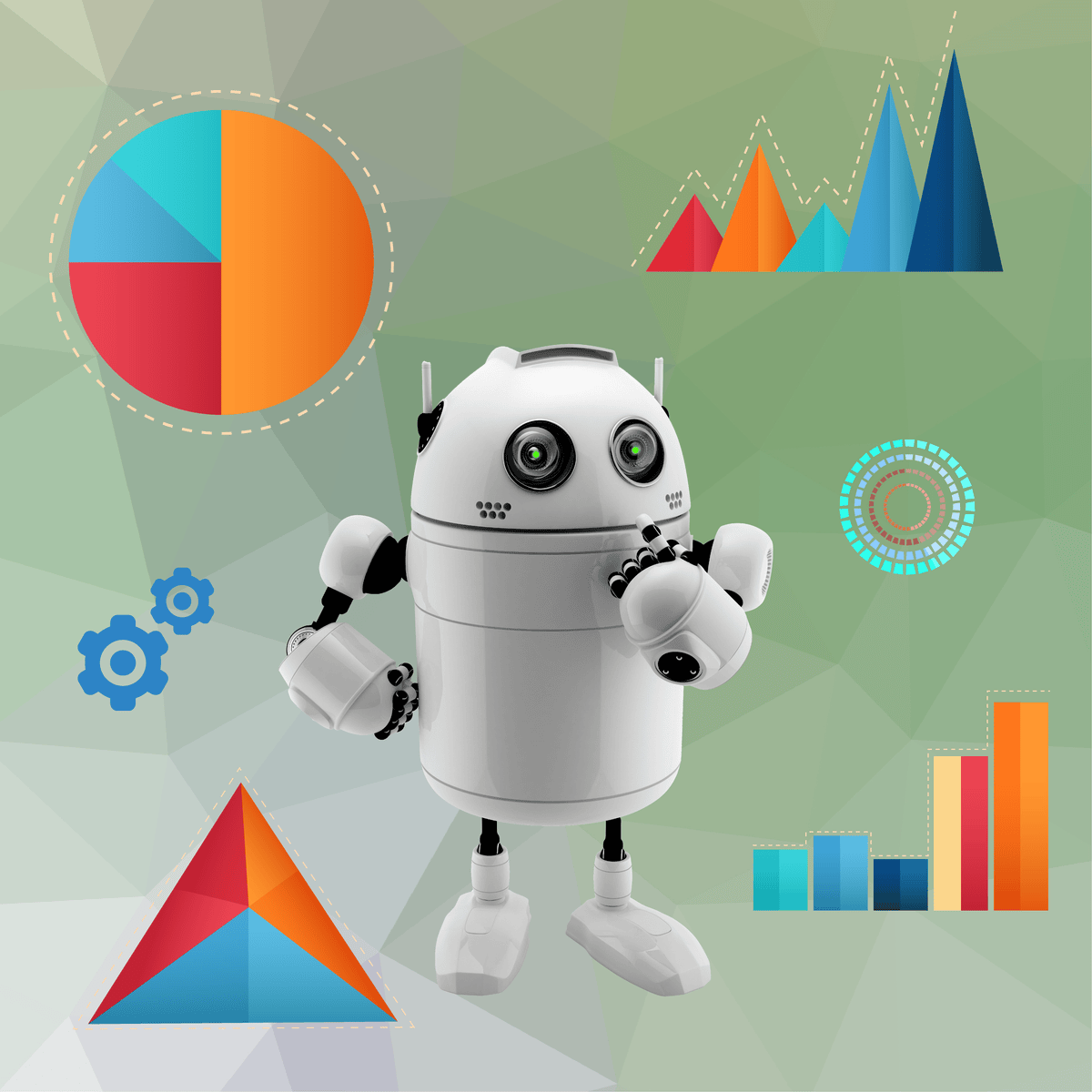
Transcript
How do we get or acquire data, and how could generative
AI improve this process? We sometimes talk about
data that can be captured, like some wild animal. Sometimes we talk about it being collected like a
basket of fruit, but also extracted like oil or even generated
like electricity. We can even talk
about acquiring data like some suspicious sum of
money that landed on our lap. What's important to
remember here is the concept of
recording and encoding. You're transforming
things around you into digital data by recording the information in some way and encoding it in a computer
processable format. Remembering the fact
that data isn't just passively obtained in some
pristine form should serve as a reminder that you
need to think critically about where the data comes from and what it's
supposed to represent. Data doesn't spontaneously
appear to us. We humans are involved in the
process. That's undeniable. But the way we are involved and the extent to which we
are involved is variable. It can be as direct
as counting items, like the number of empty
cups on your desk to using sophisticated tools that have automated processes
of recording data, like a heart rate monitor, or even a Geiger counter, which is an
electronic instrument used for measuring
ionizing radiation. Even things is automated as a Geiger counter or
heart rate monitor have embedded in them decisions that people made about
what to measure. Getting curious about
what it is that's being recorded and what the
potential limitations might be of the
representation that you're capturing is
something that's going to really
improve your ability to generate valuable
insights with data analysis. You might have an idea of a
question you want to ask, and GenAI can help you go
from question to data. Maybe you're interested
in why some areas of the same city are hotter than
others during the summer. You could use a text-to-text
generative AI tool like ChatGPT or Claude to
brainstorm your question, using a prompt like, I'm
interested in understanding why some areas of the same city are hotter than others
during the summer. What kind of data do I need
to answer my question? You take this as a
starting point and then prompt further
for specifics. How can I take
temperature measurements? You dig in critically to understand what
your options are. What are the alternatives? What are the advantages and disadvantages of different
methods of data collection? Depending on the
tool that you use, it may be trained on
data that can give you an answer on where to
find existing datasets. Or if it's integrated
with a web browser, it can get you that information
with a prompt like, what are some publicly
available sources of data that could help
me answer my question? You may want to play around with the terms data repositories
and data bases as well. Both are terms for
collections of data, where a data base
tends to specify usually something more
structured and specific, like an inventory system
used in a warehouse that uses a very particular
consistent format. While a data repository
can contain a wide array of structured and
unstructured collections of different types of data. For example, researchers often save things on
websites like Zanoto, where you can host images, spreadsheets, all kinds of
modalities and formats. Speaking of modalities
and formats, let's break down
those terms next.