AI/ML in Investment Management: Key Value Drivers and Pitfalls
In this video, Andrew Wu, Assistant Professor of Technology and Operations, discusses some key value drivers and pitfalls in the applications of AI and machine learning in investments.
Excerpt From
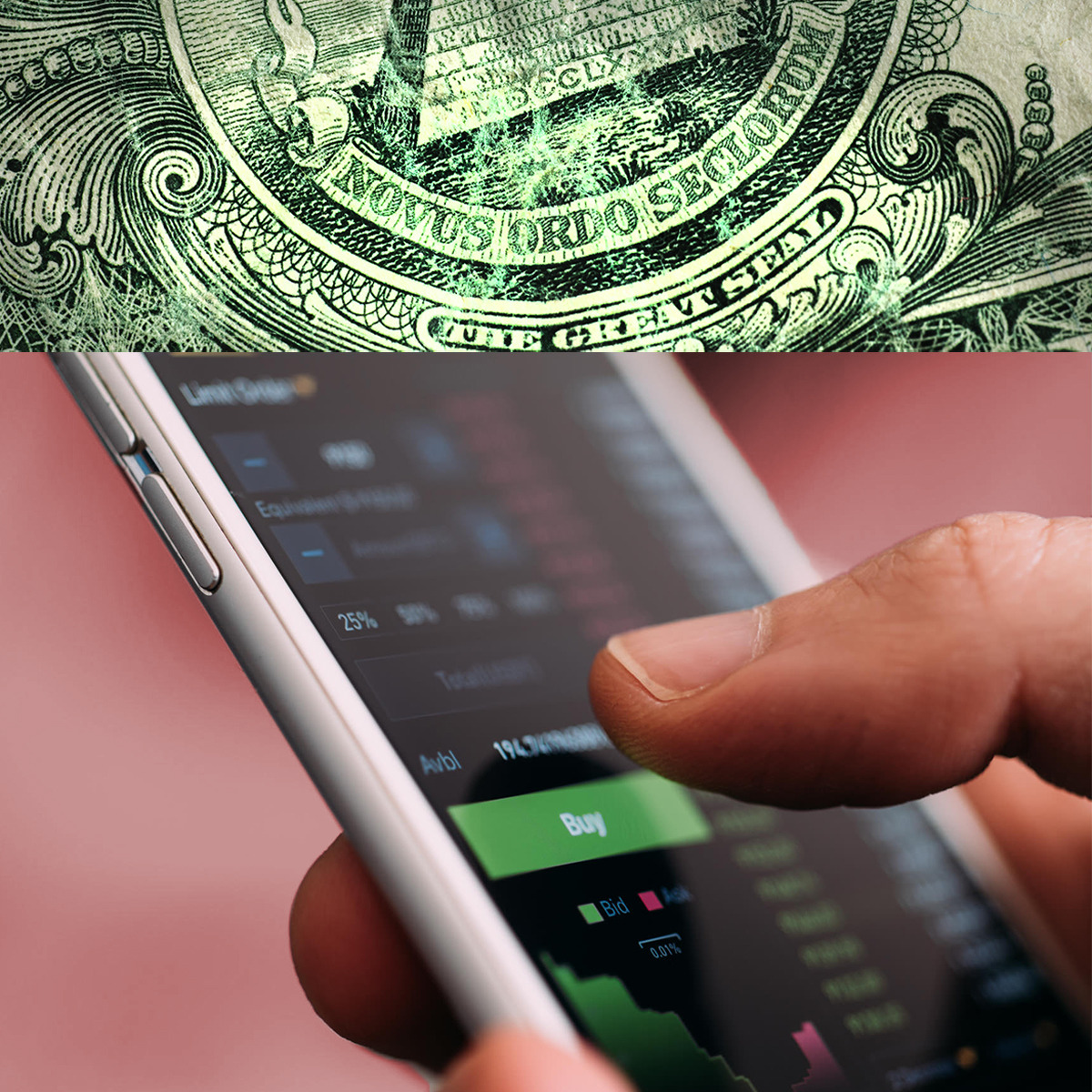
Transcript
Now that we've seen some exciting new applications of AI and machine learning in investments, it's time to introduce a word of caution and talk about some key value drivers and pitfalls in these types of applications. Particularly, if you're just getting started and aspire to be an expert in machine learning or big data in finance, these are some very common roadblocks that you should watch out for. A lot of these roadblocks can be summarized by what Sanjiv Das labeled as, "The two curses of predictive analytics." We use AI and machine learning in finance and investment to try to predict the future. Make a lot of money if you get it right. However, these tools don't really give us a crystal ball, and they have some critical limitations that you need to be aware of before trying to use them as crystal balls. The first limitation comes from non-stationarity in the data. Most of our machine learning models have an implicit stationarity assumption that the joint distribution of all the variables used in the model remains constant over time. In nontechnical terms, these models assume that the underlying regimes generating the data never change. The quality of the data you receive today is going to be the same as the quality of the data you receive tomorrow. Now we know in reality that this is not true. The data provider might change, the quality standard might be different, or there could be macroeconomic conditions like a recession that temporarily change the entire financial data landscape. Now if these changes happen, and happen often, the models that we use will become less reliable because we wouldn't be able to tell if the results that we see from the model are the result of actual useful signals or from changes in the underlying data regime. Therefore, before using machine learning models, you should investigate the underlying data sources very carefully.
The second curse, randomness, is even more problematic. Say the model deliver some results. You got it right this time. You predicted the future, and it happened to be just as you predicted. Does that mean you have a great model? Well, not necessarily because you could simply be lucky. For example, if there's a lot of noise in the underlying data, the model will give you a lot of predictions, and the more predictions it gives, the more likely that it'll happen to get some of them right, but purely by chance. If you run 10,000 regressions with all regressor combinations on even a completely random dataset, I guarantee that you'll find some significant results. But that will be meaningless because the result could be purely spurious. So the corollary here is that you should really be aware that correlation does not necessarily imply causality. The patterns that you find might be due to random chance, data regime changes, or other factors not related to your question or your model. Let me give you some examples. This is from an interesting research done at the University of Rochester. This is the conjunction between the planets Mars and Saturn. That is the relative angle of overlap between Mars and Saturn as observed on Earth. Similarly, this is the conjunction between Saturn and Jupiter. Finally, this is the number of sunspots that we see each month. The panel to the right shows that these astronomical phenomena can actually significantly, "predict," the performances of many common trading strategies like momentum, trading, size, and value, etc. But hopefully, you won't use the stars to help you time the market because again, the correlations found here are likely to be purely random. Another example is what I call the SMART Beta portfolio or S-M-A-R-T Beta portfolio. To use some common marketing language, let's construct a "Multi-factor portfolio" consisting of stocks exhibiting the following characteristics: Stocks with tickers starting with the letter S, the letter M, letter A, letter R, and letter T. Let's form the portfolio, and guess what? Between 1994 and 2013, the S-M-A-R-T Beta portfolio has outperformed the S&P almost by twice. Here again, hopefully, you see that this is also quite meaningless because I pick the period where the portfolio would exhibit the largest, "outperformance." If I shift to another period, you will have an entirely different performance result.
So the lesson here is to watch out for these spurious relations, and when you see a performance number, don't take it at face value, but really ask questions about the source of that performance, and this brings us to the lessons on key value drivers and pitfalls in applying AI and machine learning to investments. Perhaps, the most important lesson is don't use AI and machine learning tools on every piece of data just because you can use them. If you wield these tools like a big hammer and hammer everything that looks like a nail, you'll hit some nails, but the results might be spurious. So you really want to start with a question. A hypothesis about what results you expect to see and what might be driving these potential results. Only then should you ask, "Okay, what kind of data do I need to answer that question," and only then should you start looking at the tools that are best at analyzing these data. You want to have the attitude of question first, data second, and tools last. Many new investors start with the opposite order and pay for these mistakes with unnecessary losses. Once you have that attitude, you should also watch out for other potential pitfalls. Some of them we have talked about in this course. GIGO or Garbage In Garbage Out means that your result is only going to be as good as your data. If you feed your model low quality data, you're going to have a low quality result. So pay attention to your data sources. A related point is B-I-N-B or Bigger Is Not Better. Which means that instead of trying to find the biggest big data to answer your questions, you should really focus on getting the right data. Because if you collect a lot of data and not use them correctly, you'll end up having a lot of results and increase your chance of finding something spurious and being misled by them. So again, before you embark on a quest to use machine learning and big data to help with the investment process, always think about what questions you want answered, and always start with a solid economic hypothesis to support that question.