AI/ML in Investment Management: Current Applications
In this video, Andrew Wu, Assistant Professor of Technology and Operations, discusses the role of artificial intelligence and machine learning in investments. He speaks on the level of involvement that it currently has, the common issues with AI-based investing (lack of transparency, investor adoption, and data limitations), and ongoing trends.
Excerpt From
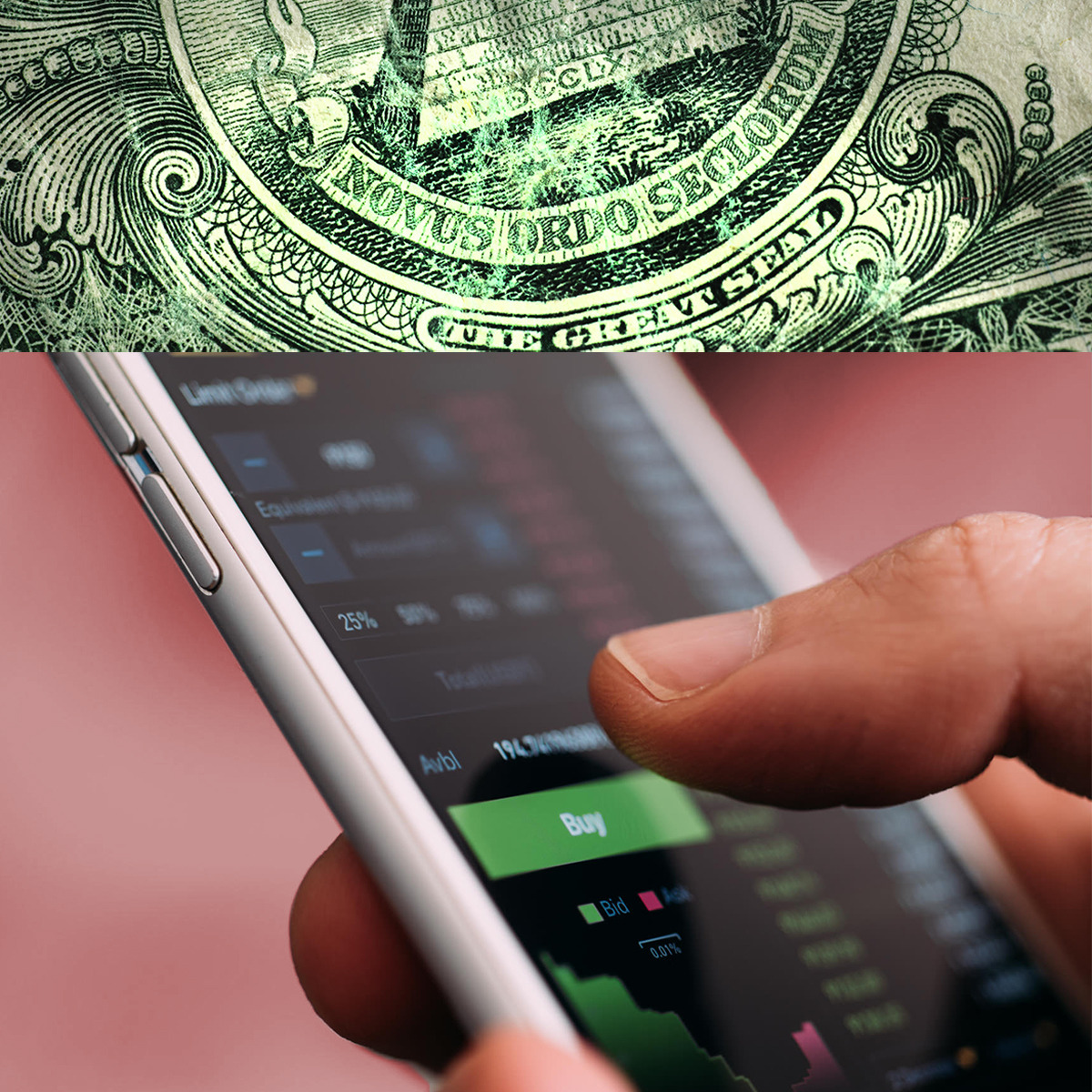
Transcript
Now that we have clarified the meaning of key concepts such as AI, deep learning, and unstructured data, let's have an overview of how they're actually applied in the business of investment management. The investment industry is undergoing a major transformation with advances in information and data analytics. And this transformation is probably even more pronounced than in the other sectors that we discussed in this fintech series like payment and lending. This is because instead of outsiders like tech startups trying to disrupt the game, investors themselves, particularly institutions such as mutual, pension, and hedge funds, are actively chasing and adopting these new technologies in the competition for market-beating returns. Consequently, we're at a stage where even tasks that are traditionally thought of as requiring a high cognitive level such as asset allocation, security selection, trading, and economic forecasting are increasingly being outsourced fully to advanced machine learning algorithms.
Think about a traditional stock trader, what image pops into your head? Well, maybe someone who's busy analyzing stock charts for things like trends, tops, bottoms, etc, right? Well, some of these tasks like chart reading can be done much more accurately and efficiently by computers. New machine learning tools like signal processing and neural networks can spot much more nuanced patterns in the data that humans cannot detect. And consequently, these high-level tasks are increasingly being done by algorithms. This is already happening in a lot of institutions like BlackRock which is going to replace more than half of its human traders with machine learning algorithms. More adventurous entities like Sentient would have hedge funds entirely run by machine learning algorithms end-to-end from stock selection to portfolio construction and to trade execution. For individuals, now they're even online, "crowdfunding" platforms like Quantopian and Numerai that aim to crowdsource new investment algorithms directly from the investing public. Like other funding platforms, they directly connect the individual traders on one side with capital providing investors on the other side. These platforms will often get their financial databases for free to the individual investors to test their algorithms on. Then, they will pull investor money to fund the algorithms that perform well in the back test.
So overall in the past decade, we saw AI and machine learning being firmly established as a powerful and necessary decision support system that help us make more informed investment decisions. And the corollary is that data, both structured and unstructured, are becoming more and more important and valuable. More complex models need more and better data to properly train and estimate. And consequently, any new data that can potentially provide extra insights are increasingly being sought after. And there are even now specialists like RavenPack that gathers data in real-time such as news and social media as soon as they came out, process these data in real-time and sell the outputs to investment institutions.
Now to the downside, there are some common issues with using so much AI and machine learning in our investment decisions. Perhaps the most important issue to be aware of is the lack of transparency. A lot of the machine learning models are well quite complex and are very difficult to understand. Even if you spend the time to understand them, the results from a lot of these algorithms are often very hard to replicate. And most investors are not willing to share their secrets. This again leads to an information asymmetry problem where you can have an institution claiming to use advanced algorithms, but in reality are doing none of that or worse. These types of AI scams are on the rise and might undermine investor confidence in AI-based investing. A related point is that because these algorithms are so complex and many are claiming to be highly automated, many investors might actually be reluctant to turn over their trading accounts completely over to these algorithms. Research has indicated that many people have an aversion to too much automation when it comes to money. "Well, are you really want my money to be managed by a robot? Robots like the ones that we saw in the movies are either dumb or evil. And I like to have some good old-fashioned human touch to it." Rational or not, these aversions might pose some roadblocks in the future growth of AI-based strategies. Finally, even if you're not afraid of AI and you can understand these algorithms, they still have a really short track record. Most of the strategies launched really in the latter half of the last decade and we're not sure if their performance can be persistent yet. To illustrate this, let's compare the performance of AI-based hedge funds with human-based funds and the broader market.
This graph uses data from Eureka Hedge's hedge fund performance indices from December 2010 to December 2019. The blue line is the cumulative performance index of AI-based hedge funds. As you can see, indeed, the AI funds have significantly outperformed the overall hedge fund index, which is the orange line below. However, the picture is a lot less rosy if we superimpose the S&P 500 Index to it. As you can see, in fact, both types of funds have underperformed the broader market. Granted, the AI fund index is a lot less volatile but depending on which period you choose is really hard to say which strategies have a consistently higher sharpe ratio. And this brings us to some predictions on what might happen in the future for the investment industry in the age of AI and machine learning. Based on the adoption trends and limitations, the near future will probably be a period of continued transformation instead of truly revolutionary changes. Barring any quantum leaps in computing, pun intended, we'll just continue our current trend. There will be more data available every day as it has been and we'll have faster computers to analyze them more efficiently. And we'll continue to bring more cutting-edge algorithms that are more adept at analyzing large-scale financial data. By the same token, analytics and automation will continue to play a more and more important role in the investment industry. And the trend of automating away more cognitive tasks will continue and probably will expand to other areas in the financial sector.
Traditional jobs that are considered "human-centric" and safe from automation like sales and marketing in banks, for example, are no longer that safe. New advancements in natural language processing, for instance, are rapidly enhancing computer's ability to generate human speech. This has already been applied in many chatterbox customer service applications and some of you might have seen those in action. But they're now spreading to even more advanced areas like writing contracts and generating analysts' reports, threatening the jobs of some securities lawyers and research analysts alike. And this trend will keep expanding and we'll see a much bigger role for data analytics, AI, and machine learning in other investment-related peripheral fields such as regulatory compliance, fraud detection, market research, and risk management.